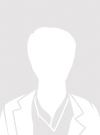
Contributions
Abstract: EP1441
Type: Poster Sessions
Abstract Category: Clinical aspects of MS - Neuro-ophthalmology
Introduction: Pupillometry measures dynamic changes in pupillary diameter in response to light stimuli, providing a functional assessment of RGC axonal pathways in the Inflammatory Demyelinating Diseases of the Central Nervous System.
Aims: This study aimed to use machine learning technique to determine if a subset of Multiple Sclerosis (MS) patients with different disease course and Neuromyelitis Optica spectrum disorders (NMOSD) may have any distinguishing pupillary characteristics.
Methods: We analyzed Pupillometry data including neurological pupil index (Npi), pupil size (PS), minimum size of pupil (MinPS), percentage change of pupil size (CH), Constriction Velocity (CV), Maximum of Constriction Velocity (MCV), Dilation Velocity (DV) and latency (LAT) from 232 patients (169RRMS-29SPMS-21PPMS-13NMOSD).In this Cohort pupillometry parameters of 90 percent of each group is used as train and 10 remaining percent is used as a test in 10 fold cross validation for predicting the type of patients.
We have used nine base learners including Linear SVM, Poly SVM, Radial SVM, random forest, decision tree, Cart tree, K Nearest Neighbors, Naïve Bayes and RPART. Majority voting on the base learners' decisions has been used to make the final decision about each sample. This ensemble learning method achieved the total accuracy of 0.75.
Results: Among all pupillary response features Latency, Constriction Velocity (CV), Maximum of Constriction Velocity (MCV), Dilation Velocity (DV) and the discrepancy between two eyes in neurological pupil index (Npi) were more discriminative than other features according to the calculated feature importance.
Conclusions: We observed that applying machine learning technique to pupillometry data has potential to yield better discrimination of group differences. Our results may help to identify a pattern of alterations in specific pupillary parameters between MS patients with different disease course and NMOSD and may assist in clinical method planning in studies targeting pupillary light reflex pathway.
Disclosure: Prof. Rieckmann has received personal compensation for consulting, serving on a scientific advisory board, speaking, or other activities with Merck, Biogen Idec, Bayer Schering Pharma, Boehringer-Ingelheim, Sanofi-Aventis, Genzyme, Novartis, Teva Pharmaceutical Industries, and Serono Symposia International Foundation.
The following authors have nothing to disclose(Roya Abolfazl, Sara Samadzadeh, Babak Khorsand, Javad Zahiri, Seyed Shahriar Arab, Siamak Najafinia, Christian Morcinek)
Abstract: EP1441
Type: Poster Sessions
Abstract Category: Clinical aspects of MS - Neuro-ophthalmology
Introduction: Pupillometry measures dynamic changes in pupillary diameter in response to light stimuli, providing a functional assessment of RGC axonal pathways in the Inflammatory Demyelinating Diseases of the Central Nervous System.
Aims: This study aimed to use machine learning technique to determine if a subset of Multiple Sclerosis (MS) patients with different disease course and Neuromyelitis Optica spectrum disorders (NMOSD) may have any distinguishing pupillary characteristics.
Methods: We analyzed Pupillometry data including neurological pupil index (Npi), pupil size (PS), minimum size of pupil (MinPS), percentage change of pupil size (CH), Constriction Velocity (CV), Maximum of Constriction Velocity (MCV), Dilation Velocity (DV) and latency (LAT) from 232 patients (169RRMS-29SPMS-21PPMS-13NMOSD).In this Cohort pupillometry parameters of 90 percent of each group is used as train and 10 remaining percent is used as a test in 10 fold cross validation for predicting the type of patients.
We have used nine base learners including Linear SVM, Poly SVM, Radial SVM, random forest, decision tree, Cart tree, K Nearest Neighbors, Naïve Bayes and RPART. Majority voting on the base learners' decisions has been used to make the final decision about each sample. This ensemble learning method achieved the total accuracy of 0.75.
Results: Among all pupillary response features Latency, Constriction Velocity (CV), Maximum of Constriction Velocity (MCV), Dilation Velocity (DV) and the discrepancy between two eyes in neurological pupil index (Npi) were more discriminative than other features according to the calculated feature importance.
Conclusions: We observed that applying machine learning technique to pupillometry data has potential to yield better discrimination of group differences. Our results may help to identify a pattern of alterations in specific pupillary parameters between MS patients with different disease course and NMOSD and may assist in clinical method planning in studies targeting pupillary light reflex pathway.
Disclosure: Prof. Rieckmann has received personal compensation for consulting, serving on a scientific advisory board, speaking, or other activities with Merck, Biogen Idec, Bayer Schering Pharma, Boehringer-Ingelheim, Sanofi-Aventis, Genzyme, Novartis, Teva Pharmaceutical Industries, and Serono Symposia International Foundation.
The following authors have nothing to disclose(Roya Abolfazl, Sara Samadzadeh, Babak Khorsand, Javad Zahiri, Seyed Shahriar Arab, Siamak Najafinia, Christian Morcinek)