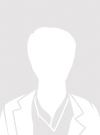
Contributions
Abstract: P1130
Type: Poster Sessions
Abstract Category: Pathology and pathogenesis of MS - MRI and PET
Background: Brain white matter lesion volume, as measured using magnetic resonance imaging (MRI), is a common biomarker in multiple sclerosis (MS). Historically, however, it has shown only modest associations with clinical outcomes. Lesion segmentations on MRI can be obtained by manual, semi-automatic, or automatic approaches. Unfortunately, several studies have documented systematic disagreement among automated approaches, especially in subjects with high lesion loads (e.g., >30mL). Using data from the 2015 Longitudinal MS Lesion Segmentation Challenge, we found that automatic approaches proposed were not immune to these biases and variability.
Objectives: We introduce a statistical technique for reducing the volumetric bias between any probabilistic lesion segmentation algorithm and a manual rater by optimizing similarity metrics among raters.
Methods: Methods were developed and assessed in 94 people with MS imaged at the Johns Hopkins Hospital (Baltimore, MD). Brain MRI was used to assess the probability of a lesion at each location using a previously published statistical segmentation algorithm. Subject-specific thresholding was applied using a novel fully automated technique, Threshold Adjustment to Probability Map Automatic Segmentations (TAPAS), to determine lesion volume. Bias reduction in volume estimates was assessed by comparing absolute error before and after TAPAS correction.
Results: TAPAS-corrected volumes and the manually acquired volumes were highly correlated (r = 0.96, P < 0.001) compared to the correlation between uncorrected volumes and manually acquired volumes (r = 0.95, P < 0.001). The average absolute error in TAPAS-corrected volumes was 2.14 (SD = 0.62) mL compared to 2.79 (SD = 0.49) mL for non-corrected volumes. Correlations between each volume and clinical covariates, EDSS and disease duration, yielded similar relationships. There were no significant differences in Sorenson's dice coefficient between traditional segmentation masks and TAPAS segmentation masks.
Conclusions: This study introduces a novel technique for obtaining accurate MS brain lesion volume estimates with automatic segmentation approaches subject to bias. The proposed pipeline allows a more accurate estimation of lesion volume at the subject level, which correlated highly with manual volumes.
Disclosure: Study Support: NIH R01NS085211, R21NS093349, R01MH112847, R01NS060910, R01EB017255, R01NS082347, R01EB012547, 2R01NS060910-09A1, NIND 2037033, NMSS RG-1507-05243, and NMSS RG-1707-28586. The content is solely the responsibility of the authors and does not necessarily represent the official views of the funding agencies.
Disclosures: Ms. Valcarcel has nothing to disclose. Dr. Muschelli has nothing to disclose. Dr. Crainiceanu has nothing to disclose. Dr. Pham has nothing to disclose. Dr. Calabresi has received personal consulting fees for serving on SABs for Biogen and Disarm Therapeutics. He is PI on grants to JHU from Biogen, Novartis, Sanofi, Annexon and MedImmune. Dr. Bakshi has received consulting fees from Bayer, EMD Serono, Genentech, Guerbet, Sanofi-Genzyme, and Shire and research support from EMD Serono and Sanofi-Genzyme. Dr. Shinohara has received consulting fees from Genentech and Roche.
Abstract: P1130
Type: Poster Sessions
Abstract Category: Pathology and pathogenesis of MS - MRI and PET
Background: Brain white matter lesion volume, as measured using magnetic resonance imaging (MRI), is a common biomarker in multiple sclerosis (MS). Historically, however, it has shown only modest associations with clinical outcomes. Lesion segmentations on MRI can be obtained by manual, semi-automatic, or automatic approaches. Unfortunately, several studies have documented systematic disagreement among automated approaches, especially in subjects with high lesion loads (e.g., >30mL). Using data from the 2015 Longitudinal MS Lesion Segmentation Challenge, we found that automatic approaches proposed were not immune to these biases and variability.
Objectives: We introduce a statistical technique for reducing the volumetric bias between any probabilistic lesion segmentation algorithm and a manual rater by optimizing similarity metrics among raters.
Methods: Methods were developed and assessed in 94 people with MS imaged at the Johns Hopkins Hospital (Baltimore, MD). Brain MRI was used to assess the probability of a lesion at each location using a previously published statistical segmentation algorithm. Subject-specific thresholding was applied using a novel fully automated technique, Threshold Adjustment to Probability Map Automatic Segmentations (TAPAS), to determine lesion volume. Bias reduction in volume estimates was assessed by comparing absolute error before and after TAPAS correction.
Results: TAPAS-corrected volumes and the manually acquired volumes were highly correlated (r = 0.96, P < 0.001) compared to the correlation between uncorrected volumes and manually acquired volumes (r = 0.95, P < 0.001). The average absolute error in TAPAS-corrected volumes was 2.14 (SD = 0.62) mL compared to 2.79 (SD = 0.49) mL for non-corrected volumes. Correlations between each volume and clinical covariates, EDSS and disease duration, yielded similar relationships. There were no significant differences in Sorenson's dice coefficient between traditional segmentation masks and TAPAS segmentation masks.
Conclusions: This study introduces a novel technique for obtaining accurate MS brain lesion volume estimates with automatic segmentation approaches subject to bias. The proposed pipeline allows a more accurate estimation of lesion volume at the subject level, which correlated highly with manual volumes.
Disclosure: Study Support: NIH R01NS085211, R21NS093349, R01MH112847, R01NS060910, R01EB017255, R01NS082347, R01EB012547, 2R01NS060910-09A1, NIND 2037033, NMSS RG-1507-05243, and NMSS RG-1707-28586. The content is solely the responsibility of the authors and does not necessarily represent the official views of the funding agencies.
Disclosures: Ms. Valcarcel has nothing to disclose. Dr. Muschelli has nothing to disclose. Dr. Crainiceanu has nothing to disclose. Dr. Pham has nothing to disclose. Dr. Calabresi has received personal consulting fees for serving on SABs for Biogen and Disarm Therapeutics. He is PI on grants to JHU from Biogen, Novartis, Sanofi, Annexon and MedImmune. Dr. Bakshi has received consulting fees from Bayer, EMD Serono, Genentech, Guerbet, Sanofi-Genzyme, and Shire and research support from EMD Serono and Sanofi-Genzyme. Dr. Shinohara has received consulting fees from Genentech and Roche.