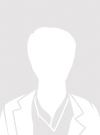
Contributions
Abstract: P584
Type: Poster
Abstract Category: Pathology and pathogenesis of MS - 21 Imaging
Background: Magnetic resonance imaging (MRI) is a critical tool for the diagnosis and ongoing investigation of multiple sclerosis (MS). Manual analysis of MRI data, however, is expensive and time consuming. Automated processing significantly reduces the amount of time and manual interaction that is required in the processing of MRI data. New methods for automated processing are introduced in the literature constantly, but these are often difficult to interchange in a large-scale processing pipeline. We developed a modular, automated framework, BRAINMAP (Bridging Radiology And Investigative Neurology using Modular, Automated Pypelines), which we implemented for the analysis of a longitudinal MS study.
Objective: To implement and apply BRAINMAP to a large-scale, longitudinal MS study, and determine rates of failure.
Methods: 166 MS patients (740 MRI scans) underwent MRI as a part of a longitudinal study (mean of 5.4 annual visits). BRAINMAP was implemented in Python using Nipype and executed in parallel on a high-performance supercomputing cluster. All images within a time point were coregistered to the time point T1-weighted image and then registered longitudinally to the baseline for analysis. Multi-Contrast Brain Stripping Method was used for skull removal, Subject-Specific Sparse Dictionary Learning for lesion segmentation, Multi-Atlas Cortical Reconstruction Using Implicit Surface Evolution for brain segmentation and Random Forest Thalamus Segmentation for refined thalamic segmentation. Lesions were inpainted for later analysis. Results underwent manual quality assurance (QA). Global atrophy was calculated on subjects that had at least 2 time points, after removal of failed sessions.
Results: After QA, 13 scans were removed due to excessive artifacts, as noted by raters. Of the remaining 727 scans, BRAINMAP passed QA on 699 scans (96.1%). Of the 28 failed scans, 17 (2.3%) failed in skull removal, 2 (0.2%) failed in brain segmentation, and 4 (0.4%) had gross lesion segmentation errors, after visual inspection. Global atrophy was measured at an annual rate of -0.45% for the whole brain, -0.50% for the cortical grey matter (CGM), -0.41% for the WM and -0.69% for the thalamus. All rates were statistically significant with p< 0.0001.
Conclusion: BRAINMAP completed with a 96.1% success rate. Statistically significant atrophy in CGM and thalamus was observed, as expected, showcasing the ability of automated processing to produce quality research results.
Disclosure:
Blake Dewey: Nothing to disclose
Natalia Caldito: Nothing to disclose
Elias Sotirchos: Nothing to disclose
Jeffery Glaister: Nothing to disclose
Kate Fitzgerald: Nothing to disclose
Aaron Carass: Nothing to disclose
Shiv Saidha: Shiv Saidha has received consulting fees from Medical Logix for the development of CME programs in neurology, consulting fees from Axon Advisors LLC, speaking honoraria from the National Association of Managed Care Physicians, Family Medicine Foundation of West Virginia, and Advanced Studies in Medicine and served on scientific advisory boards for Biogen-Idec, Genzyme, Genentech Corporation & Novartis. He receives research support from Genentech Corporation and the National MS Society, and received support from the Race to Erase MS foundation. He is a member of the working committee of the International Multiple Sclerosis Visual System (IMSVISUAL) Consortium.
Dzung Pham: Nothing to disclose
Peter van Zijl: Dr. van Zijl is a paid lecturer for Philips Medical Systems. This arrangement has been approved by Johns Hopkins University in accordance with its conflict of interest policies.
Jerry Prince: Nothing to disclose
Peter Calabresi: Peter Calabresi has received personal consulting fees from Biogen. Peter Calabresi is PI on grants to JHU from MedImmune, Annexon, Teva, Novartis, Genzyme and Biogen.
Abstract: P584
Type: Poster
Abstract Category: Pathology and pathogenesis of MS - 21 Imaging
Background: Magnetic resonance imaging (MRI) is a critical tool for the diagnosis and ongoing investigation of multiple sclerosis (MS). Manual analysis of MRI data, however, is expensive and time consuming. Automated processing significantly reduces the amount of time and manual interaction that is required in the processing of MRI data. New methods for automated processing are introduced in the literature constantly, but these are often difficult to interchange in a large-scale processing pipeline. We developed a modular, automated framework, BRAINMAP (Bridging Radiology And Investigative Neurology using Modular, Automated Pypelines), which we implemented for the analysis of a longitudinal MS study.
Objective: To implement and apply BRAINMAP to a large-scale, longitudinal MS study, and determine rates of failure.
Methods: 166 MS patients (740 MRI scans) underwent MRI as a part of a longitudinal study (mean of 5.4 annual visits). BRAINMAP was implemented in Python using Nipype and executed in parallel on a high-performance supercomputing cluster. All images within a time point were coregistered to the time point T1-weighted image and then registered longitudinally to the baseline for analysis. Multi-Contrast Brain Stripping Method was used for skull removal, Subject-Specific Sparse Dictionary Learning for lesion segmentation, Multi-Atlas Cortical Reconstruction Using Implicit Surface Evolution for brain segmentation and Random Forest Thalamus Segmentation for refined thalamic segmentation. Lesions were inpainted for later analysis. Results underwent manual quality assurance (QA). Global atrophy was calculated on subjects that had at least 2 time points, after removal of failed sessions.
Results: After QA, 13 scans were removed due to excessive artifacts, as noted by raters. Of the remaining 727 scans, BRAINMAP passed QA on 699 scans (96.1%). Of the 28 failed scans, 17 (2.3%) failed in skull removal, 2 (0.2%) failed in brain segmentation, and 4 (0.4%) had gross lesion segmentation errors, after visual inspection. Global atrophy was measured at an annual rate of -0.45% for the whole brain, -0.50% for the cortical grey matter (CGM), -0.41% for the WM and -0.69% for the thalamus. All rates were statistically significant with p< 0.0001.
Conclusion: BRAINMAP completed with a 96.1% success rate. Statistically significant atrophy in CGM and thalamus was observed, as expected, showcasing the ability of automated processing to produce quality research results.
Disclosure:
Blake Dewey: Nothing to disclose
Natalia Caldito: Nothing to disclose
Elias Sotirchos: Nothing to disclose
Jeffery Glaister: Nothing to disclose
Kate Fitzgerald: Nothing to disclose
Aaron Carass: Nothing to disclose
Shiv Saidha: Shiv Saidha has received consulting fees from Medical Logix for the development of CME programs in neurology, consulting fees from Axon Advisors LLC, speaking honoraria from the National Association of Managed Care Physicians, Family Medicine Foundation of West Virginia, and Advanced Studies in Medicine and served on scientific advisory boards for Biogen-Idec, Genzyme, Genentech Corporation & Novartis. He receives research support from Genentech Corporation and the National MS Society, and received support from the Race to Erase MS foundation. He is a member of the working committee of the International Multiple Sclerosis Visual System (IMSVISUAL) Consortium.
Dzung Pham: Nothing to disclose
Peter van Zijl: Dr. van Zijl is a paid lecturer for Philips Medical Systems. This arrangement has been approved by Johns Hopkins University in accordance with its conflict of interest policies.
Jerry Prince: Nothing to disclose
Peter Calabresi: Peter Calabresi has received personal consulting fees from Biogen. Peter Calabresi is PI on grants to JHU from MedImmune, Annexon, Teva, Novartis, Genzyme and Biogen.